Table of content
There are many buzzwords related to data management; the most recurring ones are data lake and data warehouse. This blog covers the unique features, key differences, and contemporary trends related to these terminologies. Let’s discuss what they offer and how they work.
Data Lake
A data lake is a highly scalable storage space mainly occupied by large volumes of raw data in its primitive form until it is called for a process. Data in lake data comes from various sources that comprise a combination of clustered or organized formats and are stored with a flat architecture in different file sizes. For organizations that need to collect and store a lot of data but do not find it necessary to process and analyze it instantaneously, a data lake serves as an effective repository that provides large storage spaces quickly without any need for data being transformed.
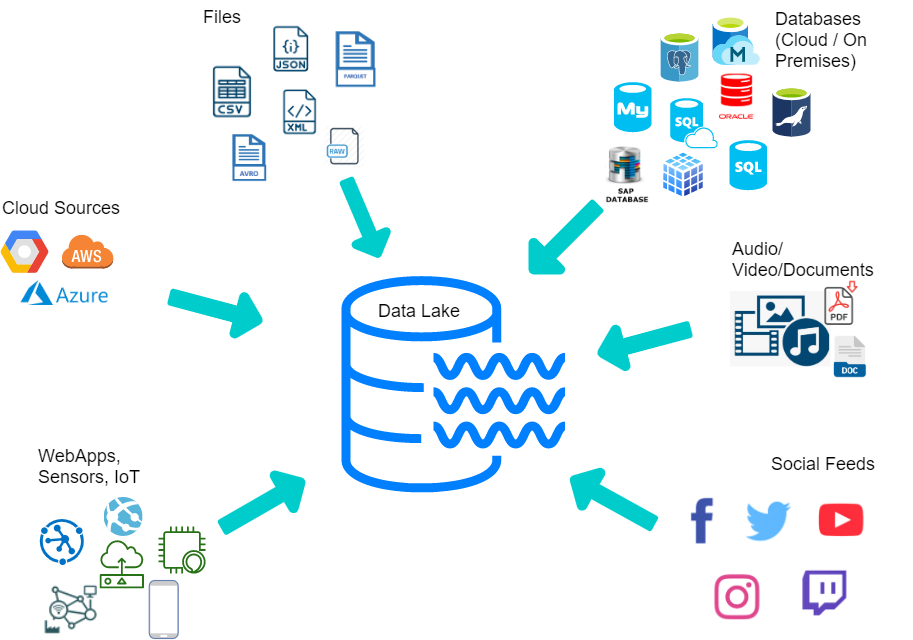
Data Warehouse
Traditional data warehouses collect and manage data for further usage in a more structured ecosystem. It performs data to information transition and provides meaningful business insights. Businesses that use data warehouses learn and analyze from their data to perform data-driven management and operational decisions.

Ref: N-ix
Also Read: ETL Pipeline and Data Pipeline – How to create an ETL Process
Differences Between Data Lake & Data Warehouse
Due to the more flexible and scalable nature, data lakes are usually considered complementary solutions to data warehouses. But both technologies have their unique features and limitations.Below are the key differences between a data lake and a data warehouse.
Layout
Raw data is data that waits to be processed for further usage. The main difference between data lakes and data warehouses is their ability to deal with raw or processed data. Data lakes primarily store raw and unprocessed data. On the other hand, data warehouses store processed and refined data.Because of this notable difference, data lakes require a much larger storage capacity than data warehouses. Secondly, raw & unprocessed data is much elastic and can be quickly called for an analysis of any kind, making it ideal for machine learning.The ability to store the raw data comes with the curse of data swamps due to the lack of appropriate quality check measures active onboard. To address this problem, data warehouses, by storing only processed and useful data save too much storage space by eliminating the portion of data that can be considered junk.
Purpose
The purpose of independent and disconnected data pieces in a data lake is not determined. Raw data is being pushed into a data lake, sometimes with predetermined future use and sometimes to store for the sake of nothing. This unfiltered data inflow makes data lakes less organized than its opponent.Since data warehouses only store processed data, all of the data in a data warehouse has been stored for a determined purpose and use within the organization. This means that storage space is not wasted on unidentified or useless data junk.
Users
Data lakes are often difficult to navigate by immature staff with less or no experience dealing with unprocessed data. Raw, unstructured data usually demands the role of a data scientist and specialized tools to transform and translate it for a useful business purpose.Processed data can be represented through bar diagrams, graphs, spreadsheets, tables, etc. This makes it understandable by most employees at a company. As we have discussed earlier, this processed data is handled by data warehouses.
Accessibility
Accessibility directly depends on how easy it is to use and access the whole data repository, not the data within. Data in Data lake architecture is stored unstructured and unconnected, which makes Data Lake easier to access. Secondly, any changes made to the data can be done instantaneously since data lakes have very few limitations and no data connections. But this environment can lead to issues like data redundancy.To overcome the issue of data redundancy, data warehouses are designed to be more structured, protected, and secure. But the strictness of structure and management controls makes data warehouses difficult and costly to manipulate as every intended change without a structured & directional mechanism is considered a violation or breach of management measures and demands expertise to manipulate.
Contemporary & Future Trends
Instead of serving as a single source of the data, the data lake provides an adaptable ecosystem that holds a variety of data, with the ability to evolve in accordance with the open access data libraries. With scalability and flexibility preferred over management and control, the data lake is made to ensure cloud storage’s core values and capabilities.As data consumers refine and analyze data, the patterns and insights they find can be pushed back into the data lake, so they are readily available to other data consumers, thus creating an ocean of data and data analytics that has never been experienced before.
This critical feedback loop makes the data lake better and easy to utilize by data consumers.Data Architecture once only portrays an ideal data warehouse, but now the cloud opens up new windows for short-lived data warehousing. A database or visualization tool is not mandatory with methodologies that can call or retrieve data from Data Lake directly.Both technologies are unique in offering their services as Data Lake is more suitable for implementing business intelligence, and data warehouse houses more managed and structured data. The critical question is not what to use but how to extract meaning and insights from data to drive a directed and fruitful business process. As data volume increases with the every day passing, so is the complexity of dealing with it, whether it stores in a lake or a warehouse.
Conclusion
The data warehouse stands as a logical representation of refined and filtered data that almost all employees in a business can use to make decisions at different levels. Without a data warehouse, decision-makers have to make a blind and slow decision that results in a business model, more vulnerable to error and mistakes.But as the amount of structured and unstructured data increases, businesses need to deploy a data lake to entertain a vast ocean of data. The contents and layout of the data lake can be determined by the nature and size of data that cannot be behold using the mainstream data warehouse.
Using both technologies, the organizations create a Business Intelligence ecosystem, a more logical model that is a data warehouse to process and manage the data with several other data visualization tools and technologies, including a data lake in parallel to increase storage scalability. In this scenario, the data lake and the conventional data warehouse work side by side to deliver fruitful results and work together as components of the larger, integrated, and more connected BI ecosystem, which in turn, add value to the data stock by delivering insights and enabling experts to make precise decisions & predictions, previously impossible.